Kaggle
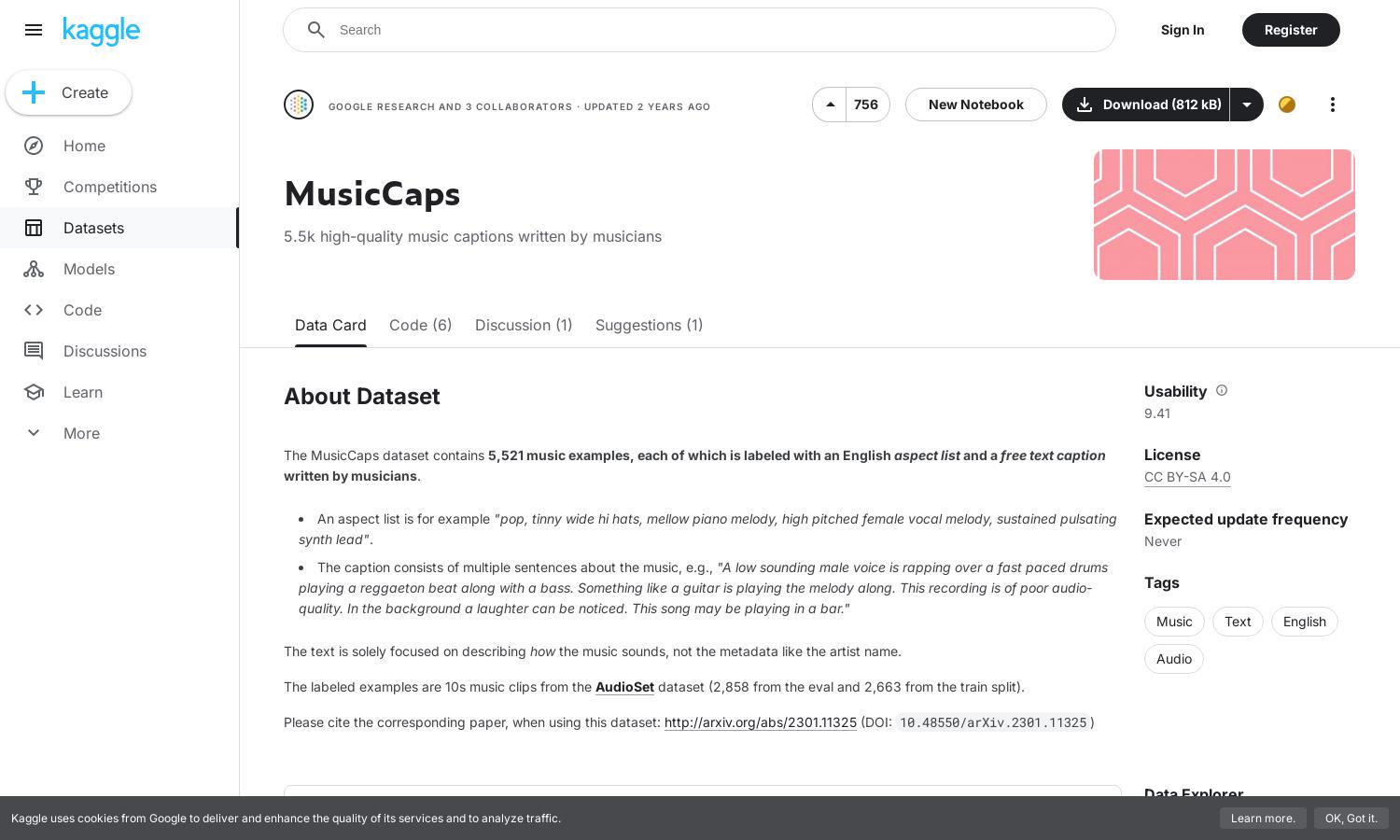
About Kaggle
Kaggle is an innovative platform that provides the MusicCaps dataset, featuring over 5,500 music captions written by musicians. Targeting researchers and developers, it allows users to explore audio descriptions and leverage high-quality data to enhance machine learning models focused on music analysis and audio understanding.
Kaggle offers free access to the MusicCaps dataset, allowing users to download the high-quality music captions without subscription fees. Users can maximize their experience with this dataset by utilizing it for research or the development of audio processing models without additional burdens or costs.
The user interface of Kaggle is designed for seamless navigation, allowing users to easily browse the MusicCaps dataset. With a clean, organized layout, Kaggle promotes efficient data exploration and provides helpful features, ensuring an enjoyable experience while working with the MusicCaps dataset.
How Kaggle works
To interact with Kaggle, users first register an account, then navigate to the MusicCaps dataset page. From there, they can explore the dataset documentation, view examples, and download the music captions as needed. The platform is user-friendly, facilitating easy access to high-quality audio and text data for various applications.
Key Features for Kaggle
High-Quality Music Captions
The MusicCaps dataset on Kaggle provides a unique collection of over 5,500 high-quality music captions written by musicians. This feature enhances the dataset's value for those studying audio processing or developing models for music analysis, offering rich insights into the audio experience.
English Aspect Lists
Kaggle's MusicCaps dataset includes detailed English aspect lists for each music example, adding depth to the audio descriptions. This feature enables researchers and developers to better analyze music elements, ensuring a comprehensive understanding of the audio content and its characteristics.
User Focused Descriptions
MusicCaps showcases user-focused audio descriptions, emphasizing how music sounds rather than metadata. This unique attribute allows users to deeply explore the auditory experience, making the dataset particularly valuable for machine learning projects in music recognition and analysis.